Document Classification
Link to Teachable Machine https://teachablemachine.withgoogle.com/models/CUO6pqBgV/
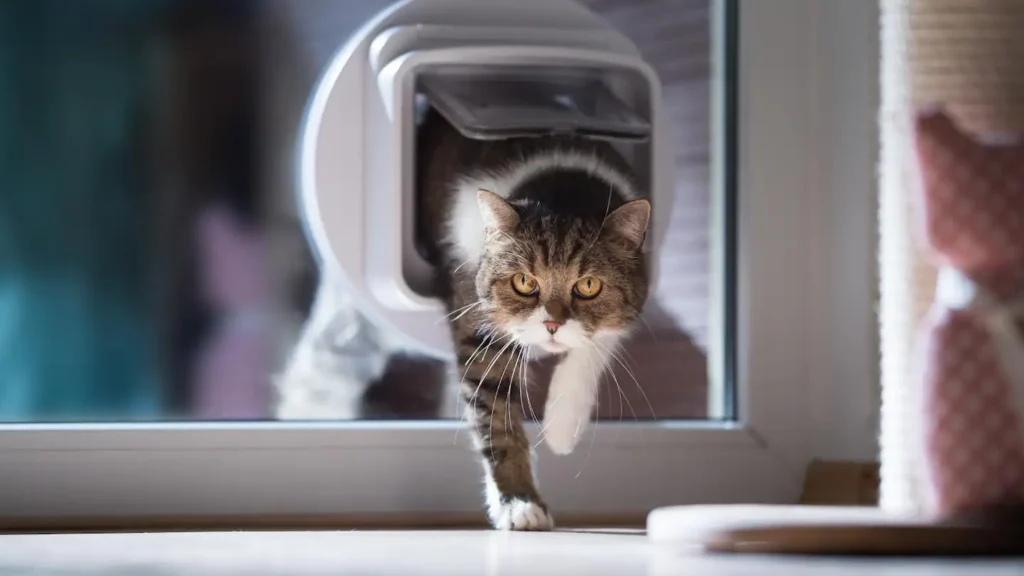
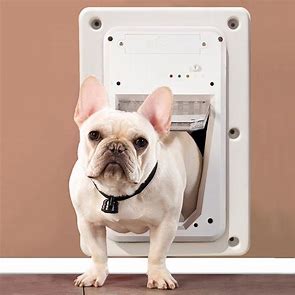
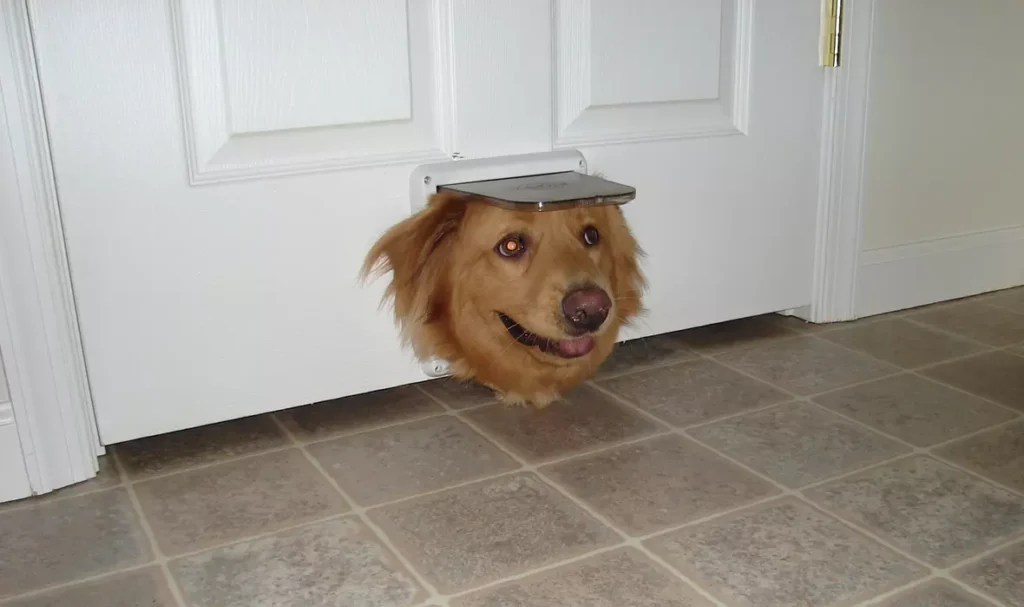
Reflection
For the special pet door project, we utilized a teachable machine to train a machine-learning model to distinguish between cats and dogs. The training dataset included a diverse set of images featuring various breeds and sizes of cats, along with images of other animals commonly found in neighborhoods, such as dogs. We chose this dataset to ensure that the model could accurately identify cats while minimizing false positives for other animals. Additionally, we included images with different backgrounds, lighting conditions, and angles to enhance the model’s build.
However, in the short term, obtaining a large dataset of labeled images can be challenging and time-consuming. While converting YouTube videos into clips could provide a larger dataset, it might also introduce noise and irrelevant information, potentially hindering the model’s performance. Our model performed well in distinguishing cats from dogs in most instances, but it might struggle with images featuring multiple animals or obscured views. Additionally, variations in lighting conditions and camera angles could also pose challenges to the model’s accuracy. Continuous refinement of the dataset and model training could address these limitations over time.